The role of data analytics and AI/ML in optimizing data center performance and efficiency
Data centers have emerged as a crucial component of the IT infrastructure of businesses. They handle vast amounts of data generated by various sources, and over the years have transformed into massive and complex entities. Of late, data analytics has emerged as a necessary ally for data center service providers, powered by the growing need to improve parameters like operational efficiency, performance, and sustainability. In this blog, we will discuss the different ways in which data analytics and AI/ML can help enhance data center management and empower data center service providers to deliver better service assurance to end-customers.
How data analytics and AI/ML can help service providers in data center optimization
Today, data center service providers are leveraging data analytics in various ways to optimize data center operations, reduce costs, enhance performance, reliability and sustainability, and improve service quality for customers. They employ a variety of methods to collect data from colocation, on-premise and edge data centers, which include physical RFID/EFC sensors, server, network and storage monitoring tools, security information and event management (SIEM) systems, configuration management databases (CMDBs), API integration, and customer usage data. The data collected is then fed into a centralized monitoring and analytics platform, which uses visualization tools, dashboards, and alert systems to analyze the data and generate insights.
Furthermore, by integrating IoT and AI/ML into data center operations, service providers are gaining deeper insights, automating various processes, and making faster business decisions. One of the most critical requirements today is for analytical tools that can help with predictive assessment and accurate decision-making for desired outcomes. This is achieved by diving deep into factors such as equipment performance, load demand curve, overall system performance, as well as intelligent risk assessment and business continuity planning. Selection of the right tools, firmware, and application layer plays a major role in making such an AI/ML platform successful.
The relationship between analytics and automation from the perspective of data centers is rather symbiotic. Data centers are already automating routine tasks such as data cleaning, data transformation, and data integration, helping data center service providers free up resources for more strategic analytics work, such as predictive modeling, forecasting, and scenario planning. In turn, data analytics provides valuable insights that enable data centers to implement intelligent automation and optimization techniques. This may include workload balancing, dynamic resource allocation, and automated incident response.
Here are some of the key areas where data analytics and automation have a significant impact:
- Enhancing operational reliability: Data analytics, AI/ML and automation can enable data centers to ensure optimal performance. This involves using predictive maintenance, studying equipment lifecycles for maintenance, and incident history analysis to learn from past experiences. In addition, AI/ML-driven vendor performance evaluation and SLA management incorporating MTTR and MTBF further strengthen operations. Leveraging these metrics within the ITIL framework helps data centers gain valuable operational insights and maintain the highest levels of uptime.
- Performance efficiency: Data centers consume a substantial amount of energy to power and maintain desirable operating conditions. To optimize services, track hotspots, prevent hardware failure, and improve overall performance, modern data centers analyze data points such as power usage, temperature, humidity, and airflow related to servers, storage devices, networking equipment, and cooling systems. Prescriptive analytics can take this a step further by providing recommendations to optimize utilization and performance.
- Predictive maintenance: Predictive analytics is a powerful technology that uses data to forecast future performance, identify and analyze risks and mitigate potential issues. By analyzing sensor data and historical trends, data center service providers can anticipate potential hardware failures and perform maintenance before they escalate, with advanced predictive analytics enabling them to improve equipment uptime by up to 20%.
- Capacity planning: Businesses today must be flexible enough to accommodate capacity changes within a matter of hours. Data center service providers also need to understand current usage metrics to plan for future equipment purchases and cater to on-demand requirements. Data analytics helps in optimizing the allocation of resources like storage, compute, and networking, while meeting fluctuations in customer needs and improving agility.
- Security and network optimization: Data centers can use analytics to monitor security events and detect vulnerabilities early to enhance their security posture. By analyzing network traffic patterns, data analytics tools help identify unusual activities that may indicate a security threat. They can also monitor network performance, identify bottlenecks, and optimize data routing.
- Customer insights: Data centers collect usage data, such as the number of users, peak usage times, and resource consumption, to better understand customer needs and optimize services accordingly. Analytics helps providers gain insights into customer behavior and needs, enabling them to build targeted solutions that offer better performance and value. For example, through customer-facing report generation, organizations and end-customers can gain valuable insights and optimize their operations. Additionally, analytics accelerates the go-to-market process by providing real-time data visibility, empowering businesses to make informed decisions quickly and stay ahead of the competition.
- Environment sustainability & energy efficiency: Data centers have traditionally consumed significant power, with standalone facilities consuming between 10-25 MW per building capacity. However, modern data center IT parks now boast capacities ranging from 200-400+ MW. This exponential growth has led to adverse environmental impacts, such as increased carbon footprint, depletion of natural resources, and soil erosion. Using AI/ML, performance indicators like CUE (Carbon Utilization Effectiveness), WUE (Water Utilization Effectiveness), and PUE (Power Utilization Effectiveness) are analyzed to assess efficiency and design green strategies, such as adopting renewable energy, implementing zero water discharge plants, achieving carbon neutrality, and using refrigerants with low GHG coefficients. For example, AI/ML modeling can help data centers achieve 8-10% saving on PUE below design PUE – helping to balance environmental impact with efficiency better than what was originally planned.
- Asset and vendor performance management: The foundation of the AI/ML platform lies in the CMDB, which comprises crucial data, including asset information, parent-child relationships, equipment performance records, maintenance history, lifecycle analysis, performance curves, and end-of-life tracking. These assets are often maintained by OEMs or vendors to ensure reliability and uptime. AI/ML aids in developing availability models that factor in SLA and KPI management. It can provide unmatched visibility into equipment corrections, necessary improvements, and vendor performance. It can also help enhance project models for expansion build-outs and greenfield designs, accurately estimating the cost of POD (point of delivery) design, project construction, and delivery.
- Ordering billing and invoicing: AI/ML plays a vital role in enhancing the efficiency and effectiveness of order, billing, and invoicing processes. Its impact spans various stages, starting from responding to RFPs to reserving space and power, managing capacity, providing early access to ready-for-service solutions, facilitating customer onboarding, and overseeing the entire customer lifecycle. This includes routine processes such as invoicing, revenue collection, order renewal, customer Right of First Refusal (ROFR) management, and exploring expansion options both within and outside the current facility.
Selecting the right data analytics solution
The implementation of data analytics and automation through AI/ML requires careful consideration as several parameters, such as data quality and level of expertise play a crucial role in delivering efficient end-results. To succeed, businesses need to choose user-friendly and intelligent solutions that can integrate well with existing solutions, handle large volumes of data, and evolve as needed.
At Sify – India’s pioneering data center service provider for over 22 years, we continuously innovate, invest in, and integrate new-age technologies like AI/ML in operations to deliver significant and desired outcomes to customers. We are infusing automation led by AI/ML in our state-of-the-art intelligent data centers across India to deliver superior customer experiences, increased efficiency, and informed decision-making, resulting in more self-sustaining and competitive ecosystems. For example, leveraging our AI/ML capabilities has been proven to lead to over 20% improvement in project delivery turnaround time. Our digital data center infrastructure services offer real-time visibility, measurability, predictability, and service support to ensure that our customers experience zero downtime and reduced Capex/Opex.
How do Sify’s AI-enabled data centers impact your business?
- Person-hour savings: Automation of customer billing data and escalations resulting in up to 300 person-hour savings in a month.
- Reduction in failures: Predictive approach for maintenance and daily checks yielding up to 20% reduced MTBF, 10% improved MTTR, and 10% reduction in unplanned/possible downtime.
- Cost savings: Improved power/rack space efficiency and savings on penalties to deliver up to 8% reduction in customer penalties by maintaining SLAs and 10% reduction in operating cost.
- Compliance adherence: Meeting global standards and ensuring operational excellence and business continuity.
To know more about our world-class data centers and how they help enterprises expect positive business outcomes, visit here.
Blue Brain – a tool or a crutch for humanity ?
What if human beings could better their brain, built across millennia through evolution? Gourav looks at the possibility of just such a technology and its implications.
We all think, act, react, ponder, decide, and memorize with the help of our brains. It is a very intriguing, interesting, and exciting part of our human body and contributes drastically to our human ecosystem.
It is also still a mystery as to how our brain, one of the most complex systems found in nature, functions.
Imagine an artificial copy of our human brain that can do the same without our help. If such a machine is created, then the boundaries between a human and a machine would grow thinner bringing to the fore its advantages and disadvantages.
The Brain and Mind Institute of École Polytechnique Fédérale de Lausanne in Switzerland did exactly that when they thought up a project called Blue Brain Project aimed at creating an artificial brain. This project was founded by Henry Markram in 2005.
What is Blue Brain Project?
The Blue Brain Project is bleeding-edge technology research that aims to reverse engineer a typical human brain into a computer simulation. Blue Brain can think, act, respond, make quick decisions, and keep anything and everything in its memory.
It means that a computer can act as a human brain taking artificial intelligence sky high. The simulations are carried out on IBM’s Blue Gene supercomputer, hence the term Blue Brain.
Why do we need this?
Today, we function based on our brain’s capability to respond to different situations. Some people make intelligent decisions and take actions as they have an inborn quality of intelligence. But this intelligence dies when we die. Imagine if such intelligence can be preserved to help the future generation.
A virtual or an artificial brain that can provide the required solution for the stated problem. Our brain tends to forget trivial things that mean more like birthdays, names of people, etc.
Such a brain can help us by storing this information and aiding whenever necessary. Imagine uploading ourselves onto a computer and living inside it.
How can this be made possible?
The information about the brain needs to be uploaded into the supercomputer to perform like a brain. So, retrieval or studying of this information is paramount. This can be made possible by using small robots called nanobots.
These bots can travel between our spine and brain to collect important data. These data contain necessary information such as the structure of the human brain, its current state, etc.
A human brain takes inputs from the sensor throughout the body, and it interprets these inputs to store in the memory or to respond to the desired output.
The artificial brain does a similar job by taking inputs from a sensory chip and it interprets these inputs by associating the input with the value stored in one of its registers which corresponds to different states of the brain.
The Blue Brain Project – Software Development Kit helps the users to utilize the data from the nanobots to visualize and inspect models and simulations. The SDK is a C++ library that is wrapped around Java and Python.
The Einstein Connection
When people think of genius, the list most assuredly includes Albert Einstein. For years, different scientific researchers have been trying to find the mystery behind his genius brain. Imagine if Einstein’s brain could be recreated with the help of the Blue Brain Project. Many intriguing inventions and discoveries could be made. Such intelligence would shape many generations to come.
The Blue Brain Project has many merits such as non-volatile memory that can store anything and everything permanently, and the capability to make intelligent decisions without the presence of a person. This research can help in curing a lot of psychological problems.
If such technology comes to people, they would be dependent on these systems. This can open the door wide open for hack threats which can pose a real danger to people. People might be fearful of using such technology and it can culminate into large resistance.
The Author’s Views
Intelligence is a quality that has always been associated with humans. Now artificially many intelligent systems and tools are available that aim to better people’s lives.
If Blue Brain technology reaches humans, everyone’s life will be enriched.
But people might get too dependent on this technology which will culminate in catastrophic problems for the human psyche. However, if used properly this technology can add new layers to human life than being a replacement.
Stage Gate Management – How to ensure nothing falls through the cracks in your Software Supply Chain?
Credits: Published by our strategic partner Kaiburr
As the technology leader (CDO / CIO / CTO / CISO or a VP of Technology / Engineering / DevOps / DevSecOps /Security / Compliance) you are looking to deliver your digital initiatives in a predictable manner and accelerate maturity of your software product teams while ensuring gaps are not introduced in the software supply chain.
To achieve this you need answers to the following questions:
- What is our current level of DevSecOps / DevOps maturity?
- Are we really doing the steps we set out to do across various stages of SDLC? How do we identify the tasks falling through the cracks in the software supply chain?
- What is our current level of risk on security, compliance, and quality?
- How effectively are we using the 15-20 tools procured?
Some examples of common issues in the software supply chain are:
After more than six years of R&D, Kaiburr, a low code /no code digital insight platform, is solving this problem meaningfully and at scale for large enterprises and top innovators. With Kaiburr, digital leaders and software teams get a single pane on their overall stage gates across the entire SDLC at the organization, business unit, portfolio, program, and product (application) level like the following:
Users can drill down on any stage gate to know specific items to be acted upon
- [ALM] Stories missing acceptance criteria or story points in tools like JIRA, Azure Boards, Gitlab
- [Source Code Mgmt.] Commits and Pull Requests missing traceability to requirements in tools like Bitbucket, GitHub
- Acceptance criteria set the bounds for the story and the scope of the work the story entails.[Code Quality] Code quality issues on features in tools like SonarQube
- [SAST] Critical static analysis vulnerabilities on the latest code merged in tools like Veracode, Checkmarx
- [SCA] Vulnerable libraries downloaded for releases in tools like Snyk, Blackduck
- [CI-CD] Build / deployment issues in tools like Jenkins, Tekton, Bamboo, Azure DevOps
- [Unit Test] Unit test coverage gaps in tools like JUnit, NUnit
- [Functional Test] Test failures in tools like Selenium, Cucumber, Katalon
- [Auto Provision] Infrastructure automation issues with tools like Terraform, Pulumi
- [Monitoring] Application monitoring issues with tools like Datadog, Dynatrace
Kaiburr adopts the following process for teams to effectively remediate gaps in the software supply chain
To add cherry on top, Kaiburr has mapped out these stage gate validations to industry standard
frameworks like NIST 800 53, CIS, ISO 27k, SOC2, GDPR, FedRAMP, HIPAA, HITRUST, PCI.
Kaiburr has deeply engineered this framework to solve this complex problem:
Software Supply Chain Challenges | How Kaiburr addresses it? |
We need to deal with multiple tools used for the same purpose. E.g., JIRA, Azure Board, Rally for ALM; Test Rail, Zephyr, HP ALM for testing | Kaiburr’s canonical models convert tool specific data to functional data. So, data from JIRA, Azure Board, Rally, Gitlab are stored in a common ALM canonical model. |
We keep migrating from one tool to another. E.g., we recently moved from Jenkins to Tekton; from Checkmarx to Veracode. | Kaiburr’s canonical models abstract tool data so will have no impact from moving to various tools. Kaiburr essentially future proofs you. |
Our processes differ between BUs, portfolios, and teams. Hence it is hard to get a standardized view across these teams. E.g., each of teams have different JIRA workflows, issue types, labels; they follow different branching strategies in github. | Kaiburr can understand different variations of processes implemented by teams in an organization and produce unified standardized output. |
We do not consistently tag our usage in various tools. Hence it is hard to know which teams are using what tools and the level of usage. | Kaiburr’s discovery engine can correlate data points and produce a linked view of events across the lifecycle for a given team, project, or initiative |
With Kaiburr
- Digital leaders can gain near real time visibility on gaps in their SDLC so they can mitigate them early in the cycle
- Developers get spoon fed on priorities so their experience and productivity is improved
- Security, Compliance and Governance leaders can identify and remediate security and compliance issues in a timely manner Digital leaders can produce audit reports on internal controls in a fully automated manner
If you want to get started with your Stage Gate Compliance journey using Kaiburr reach us at marketing@sifycorp.com
Credits: Published by our strategic partner Kaiburr
In the Kitchen and on Cloud Nine
Prashant Kanwat breaks down what a cloud kitchen is and how it is revolutionizing the online market
Gone are the days of restaurants and dining out being the only option to travel beyond the everyday home food and the kitchen. If you look around these days, seeing food aggregators such as Zomato and Swiggy running round the clock to deliver the freshest food right at the customer’s doorsteps, is a common sight.
Not only has this driven a trend among consumers, but it has also left the food entrepreneurs, the small restaurant owners, and people in the food industry, gaping at the growing trend of the online ordering business. The number of users of the online food delivery system is expected to grow up to 2.9 million users by 2026.
A cloud kitchen or a ‘ghost kitchen’ is called so, due to the physical visibility it lacks, to the public. Unlike restaurants that offer dine-in, cloud kitchens are devoid of all the setup. In fact, cloud kitchens require minimum equipment, such as space and kitchen equipment, compared to the lavish decor that restaurants use.
Types of cloud kitchen model
- Independent cloud kitchen: As the name suggests, behind the cloud kitchen is a single brand that is dependent on an online ordering system for their orders. With a small team of chefs, definitive operative hours and a brand name, independent cloud kitchens have a business model that is self-reliant and is hosted on different food aggregators to acquire customers.
- Hybrid cloud kitchen: Being a hybrid of takeaway and cloud kitchen, a hybrid cloud kitchen can be visualized as an extension of the regular cloud kitchen.
- Food aggregator owned cloud kitchen: With the aim of generating revenue and growing popularity of cloud kitchens, there are several food aggregators that lease out or purchase a convenient kitchen space to a growing food brand or one that is new in the market.
- Multi brand cloud kitchen: This cloud business model is a combination of varied brands under the same kitchen.
- Outsourced cloud kitchen: TAs the newest entry to the cloud kitchen game, this cloud kitchen business model is solely dependent on outsourcing of the food and the delivery services. A restaurant or any other business can outsource a part or the entirety of the menu such that the prepared product is received at the restaurant. The restaurant then packs the item and hands it over to the delivery personnel. The operational cost for the in-house team is reduced as everything from preparation to delivery is handled by the outsourced group.
How to set up a cloud kitchen in India
- Choosing the right rental space
- Licenses and trademark registration
Some of the licenses to procure before starting out with a cloud kitchen business model include,
- GST (Goods and Services Tax) (Goods and Services Tax) registration
- Trade license
- Fire and safety license
- FSSAI (Food Safety and Standards Authority of India) license
- Trademark registration
- Deciding the cuisine
- Kitchen space, equipment, and raw ingredients
- Online Order Management System
- Staff requirements
- Marketing
Costs associated with a cloud kitchen
The costs of setting up a cloud kitchen model in India vary depending on the city chosen, the demographics, the type of cuisine offered and so on. Here is a rough outline of the costs that might come up and a rough estimate of how much they amount to.
The resources one would have to be spending in a cloud kitchen business model include,
- Rent: This depends on the location and the land prices. A space of 600-800 sq feet is considered sufficient for a cloud kitchen model and may range from ₹25,000-50,000
- Licenses: The basic and necessary licenses cost around ₹15,000-20,000
- Staff: Having a basic set of staff can cost around ₹50,000-85,000
- Kitchen and equipment: This are solely dependent on requirement and can range from ₹5 lakh from scratch to around 8 lakhs. Basic kitchens can also be outsourced.
- Online ordering system: Many ordering systems allow customization based on features required, and these can range from ₹4,000/year to around ₹6000
- Customer acquisition and social media presence: Based on paid and organic marketing, this may cost around ₹40,000-80,000 per month
- Branding and packaging: As packaging is the crucial thing with cloud kitchen startups, branding across social media, food aggregators and effective packaging can cost around ₹50,000-70,000
Choosing the right Technology
With the right technology, you can streamline your operations and make your cloud kitchen run more smoothly. Here are a few things to keep in mind when choosing technology for your business:
- Order management system: An order management system (OMS) is a software that helps you track and manage orders. It can be used to track customer information, inventory levels, and delivery status. A good OMS will be user-friendly and scalable so that it can grow with your business.
- Kitchen display system: A kitchen display system (KDS) is a software that helps you manage food preparation and cooking. It can be used to track recipes, ingredient lists, and cook times. A good KDS will be user-friendly and customizable so that it can be adapted to your specific needs.
- Customer relationship management system: A customer relationship management (CRM) system is a software that helps you manage your relationships with customers. It can be used to track customer information, contact history, and order history. A good CRM will be user-friendly and scalable so that it can grow with your business.
- Accounting software: Accounting software is a software that helps you manage your finances. It can be used to track income, expenses, and invoices. A good accounting software will be user-friendly and customizable so that it can be adapted to your specific needs.
By investing in the right technology, you can make your cloud kitchen more efficient and organized. This will help you save time and money in the long run.
In India, the average annual cost of setting up a restaurant is almost 3x more than the set-up of a cloud kitchen, steering good entrepreneurs and food aggregators alike to jumpstart on this side of the competition.
Regardless, it is always recommended to not follow the herd and go with the requirements your business needs to succeed. Assessing market trends, costs needed, estimating the funding required, security, profitability eventually are topics to consider before getting started on a cloud kitchen model.
How Unreal and Unity are changing filmmaking
Ramji writes on the ‘Unreal Unity’ of technology and art…
The highly acclaimed Unreal and Unity3D engines are among the most popular tools with employed by the augmented Reality (AR), virtual reality (VR) and gaming professionals. But what in fact are these ‘engines’ and how is this new technology revolutionising cinema? In this article let us see what powers these new age solutions and how these technologies are changing filmmaking.
Imagine you are playing a computer game which is usually a set of sequences that appear at random, and you, the player, react or engage with them. All these happen in something called as ‘real-time’. In the computer graphics terminology, something happening in real-time means it happens instantaneously. When you are moving in the game or a VR environment, there is no way to predict what direction you would turn towards. And wherever you look within the game, there should be some visuals or environment with respective to your position. This is done by real-time rendering. Images or visuals that are produced instantly depending on the point of view. There are a lot of mathematical calculations that happen in milli or microseconds and the resultant images are shown to the user. These calculations and all other game dynamics are handled by the game engines.
Some of the popular engines right now are Unity3D and Unreal. It is interesting to see how these engines are evolving beyond the gaming industry. With realistic lighting, and almost realistic human character generators, these engines are blurring the lines between gaming and moviemaking.
For example, in the Disney+ series The Mandalorian, a novel idea called virtual production was used.
What is virtual production? This is a stage surrounded by a semi-circular LED screen on which the background or the environment is shown. The actors stand in front of the screen and enact their roles. All this while the camera records the scene with the background. This is very much like the background projections used in older movies. But the novel idea is that the backgrounds that are projected are dynamic, and the perspective will change as the camera moves. This makes the scene look realistic. And it also captures the ambient light from the background fall on the characters and the actors also know where they are located. This greatly helps in removing the usage of blue/green screen and reducing long postproduction hours.
This is how the real set and virtual set (LED Wall) is placed in the production floor. The part that is separated by the white outline is the real set with real people while the background is on the LED wall. They blend seamlessly thereby creating a continuous set.
The production team for The Mandalorian used Unreal engine to create the hyper-realistic backgrounds and these backgrounds can be changed dynamically during the filming. Using a virtual reality headset, the production team can alter the backgrounds as per the director’s vision. The real filming camera is linked to a virtual camera in Unreal engine and as the real camera moves or pans, the linked virtual camera mimics the movement thereby shifting the perspective of the (virtual) background. All these are done instantly and in “real-time”. This provides a very realistic shot, and the virtual sets can be quickly changed or altered in a jiffy!
Not only this, but there are also other dynamics like the time of the day that are made available to the filming team. They are provided by web-based controls on an iPad using REST APIs. This enables the production team to change the lighting, sky colour and time of the day all instantly. This saves a lot of time for the team and helps in improvising the shot or scene on the go.
Not the one to be left behind, Unity3D, is another popular engine that is in the fray of creating hyper-realistic movie-quality renders. They recently released a teaser called Enemies which involves completely computer-generated imagery complete with high-definition render pipeline (HDRP) for lighting, real-time hair dynamics, raytraced reflections, ambient occlusions, and global illumination. Well, these terms themselves will warrant a separate article. That’s for another day and time. Here, take a look at the teaser:
In this case, the entire shot is computer generated including the lady character. Unity3D has its own set of digital human models and Unreal has its Metahuman package that offer hyper-realistic digital characters which can be used in real-time.
This is just the tip of an iceberg. The possibilities are endless, and it is a perfect amalgamation of two fields, and this opens a lot of doors for improving filmmaking with real-time rendering technology and the line between gaming and filming are blurred by game changing technology revolutions driven by Unreal and Unity3D!
In case you missed:
- VFX – Dawn of the digital era
- VFX – The evolution
- VFX: The beginning
- Is Augmented Reality the future of retail?
- The future of training is ‘virtual’
- Putting the ‘Art’ in Artificial Intelligence!
- Into the Metaverse
How to use Acceptance Criteria to deliver high quality Product Features
Credits: Published by our strategic partner Kaiburr
As a Product Manager, when you define features for development and / or enhancement, it is important to ensure that the requirements are well-defined and unambiguous. This ensures that the product is built according to the vision and intent that you have for it.
Lack of rigorous, well-defined Acceptance Criteria can lead to delays and even poorly built products which do not answer the need for which they were intended.
What are acceptance criteria and why are they important
- Acceptance criteria in a story are the definition of ‘done’ for the story.
- They are a formal list of requirements that describe a set of features, capabilities, conditions that meet the user’s needs as defined in the story.
- Acceptance criteria set the bounds for the story and the scope of the work the story entails.
- They are a key piece of communication between the user / client / product owner and the builder / developer.
- While they do not define the implementation details and ‘how’ the story must be built, the acceptance criteria define ‘what’ requirements must be met for the story to be considered ‘done’.
- This allows the development teams to design and build the user story with a clear idea of what must be built and what must be tested.
Acceptance criteria should define:
- current or pre-existing features / functions that will be used or are assumed to already be available if applicable
- change in any existing user action / behavior
- checks on the user actions that must pass
- negative scenarios
- appropriate error handling and alerting
- outcome of user action / behavior
- key performance / speed / metric for system performance as relevant
- functions / features that are not in scope if applicable
Who defines acceptance criteria:
Usually acceptance criteria are defined by consensus. Ideally the user behavior and system performance expectation, as perceived by a user, should be defined by the Product Manager. Additional standards that must be met for performance, tracking and internal system use may be defined by the Development and Operations teams as well.
What are effective acceptance criteria:
There are several ways to define acceptance criteria and depending upon the type of product and user story, different methods may be more relevant or easier to implement.
Before jumping into the actual methods that may be used to define acceptance criteria, the following points must be kept in mind:
- Anyone who reads the acceptance criteria should be able to understand them
- Must define ‘What’ must be done not ‘How’ it must be done
- Must always be from the user’s perspective
- Must be specific, clear, concise and testable
- Must be within the scope of the story
How to define effective acceptance criteria
- Scenario-based acceptance criteria define the user journey or user experience through describing various scenarios that the user will encounter and how the experience must be handled.
Example: A user has the choice of several options for choosing and customizing a widget that we build for them: The navigation paths that are possible and allowed should be detailed.
- A picklist of standard sizes of the product
- An option to create a custom size for certain features of the widget by going to a different page on the app or browser.
- Returning to the original screen with the customization saved.
- A choice of finishes.
- A choice of delivery options.
- A choice of shipping options.
- A payment method and transaction with confirmation.
- The acceptance criteria must detail which paths are valid, which paths are complete and what happens when a path is completed, or left incomplete.
- The user may be able to save some customization to their account or profile.
- The user may be able to share the customization to external parties or not etc.
- Rule based acceptance criteria usually list a set of criteria that must be met for the story to be ‘done’. These include display fields and branding colors / logos etc. , size, appearance and shapes of visual elements.
Example: A landing page for a first time or returning user, who is requested to create an account or login with existing account:
- The logos and standard branding colors for the page or app are displayed.
- Check for existing users with email or userid as the case may be.
- Checks for password requirements for strength.
- Checks for MFA rules.
- Checks for recovery options etc.
- Custom and hybrid Rules+Scenarios used together are, not surprisingly, the most common form of defining acceptance criteria for complex product features, where both Scenarios of user experience are defined along with specific Rules and additional testable descriptive requirements.
No one way of defining criteria is better than another, and the best way is usually the one that answers all questions that any reader of the story may have, be it from the product team, development team, executive sponsor or another product and project stakeholder.
What happens if acceptance criteria are not clear or missing:
Unclear acceptance criteria can cause many headaches and derailments in the product development process:
- User requirement may be met but not in the way intended by the product manager / or described in the product roadmap.
- Testing may be successfully completed but the feature does not meet the user’s needs.
- Rework may be needed or additional requirements may be created for fixing or changing older features affected by current change.
- Rework is needed as performance metrics are poor or not met.
- Error handling for negative scenarios is ambiguous or undefined.
- Extra features / functions may be built when not needed or prioritized.
- Potential for scope creep
- Additional spending of resources – time and money – to ‘fix’ the story
Kaiburr helps identify stories missing acceptance criteria like the example below –
With just 15 minutes of configuration, Kaiburr produces real time actionable insights on end-to-end software delivery with 350+ KPIs, 600+ best practices and AI/ML models. Kaiburr integrates with all the tools used by the enterprise Agile teams to collect the metadata and generates digital insights with a sophisticated next generation business rules engine.
Reach us at marketing@sifycorp.com to get started with metrics driven continuous improvement in your organization.
Credits: Published by our strategic partner Kaiburr
eLearning Solutions to Mitigate Unconscious Hiring Bias
The Hiring Bias
In study after study, the hiring process has been proven biased and unfair, with sexism, racism, ageism, and other inherently extraneous factors playing a malevolent role. Instead of skills or experience-based recruiting, it is often the case that interviewees get the nod for reasons that have little to do with the attributes they bring to an employer.
“This causes us to make decisions in favor of one person or group to the detriment of others,” says Francesca Gino, Harvard School of Business professor describing the consequences in the workplace. “This can stymie diversity, recruiting, promotion, and retention efforts.”
Companies that adhere to principles of impartial and non-biased behavior and that want to increase workforce diversity are already hard-pressed to hire the best talent in the nation’s current environment of full-employment and staff scarcity.
Five Main Grounds for Hiring Bias
Researchers have identified a dozen or so hiring biases, starting with a recruiting ad’s phrasing that emphasizes attributes such as “competitive” and “determined” that are associated with the male gender. In fact, study findings have reiterated that even seasoned HR recruiters often fall prey to faulty associations.
Here are five of the most frequently cited reasons for the unintended bias in the hiring process:
- Confirmation Bias: Instead of proceeding with all the traditional aspects of an interview, interviewers often make up their minds in the first few minutes of talking with a candidate. The rest of the interview is then conducted in a manner to simply confirm their initial impressions.
- Expectation Anchor: In this case, interviewers get fixated on one attribute that the interviewee possess at the expense of what backgrounds and skills other applicants can bring to the interview process.
- Availability Heuristic: Although this may sound somewhat technical, all it means is that the interviewer’s judgmental attitude takes over. Examples might be the applicant’s height or weight, or something as mundane as his or her name, reminding the interviewer of someone else.
- Intuition-Based Bias: This applies to interviewers who pass judgment based on their “gut feeling” or “sixth sense”. Instead of evaluating the candidate’s achievements, this depends solely on the interviewer’s frame of mind and his or her own prejudices.
- Confirmation Bias: When the interviewer has preconceptions on significant aspects of what an applicant ought to offer, everything else gets blotted out. This often occurs when, within the first few minutes of talking with an applicant, the interviewer decides in his or her favor at the expense of everything else that other candidates may have to offer.
Why Bias Is a Problem
In a book titled The Difference: How the Power of Diversity Creates Better groups, Firms, Schools and Societies, Scot E. Page, professor of Complex Systems, Political Science and Economies at the University of Michigan, employs scientific models and corporate backgrounds to demonstrate how diversity in staffing leads to organizational advantages.
Despite the mountain of evidence, the fact remains that many fast-growing companies are still not deliberate enough in their recruiting practices, often times ending up allowing unconscious biases to permeate in their methods.
Diversity in hiring, an oft-used term, is essentially a reflection on different ways of thinking rather than on other biases. For example, a group of think-alike employees might have gotten stuck on a problem that a more diverse team might have tackled successfully using diverse thinking angles.
Automated Solutions
Although hiring bias is normally shunned, this in no way implies that it doesn’t proliferate amidst large and small organizations alike. The tech industry—and Silicon Valley in particular—was shaken recently by accusations of bias in the workplace, driving many HR managers and C-Suite executives to look for “blind” hiring solutions.
To pave the way for a more diverse workforce—one that is built purely on merit—there is recruiting software built to systematize vetting and maintain each candidate’s anonymity. These packages enable companies to select candidates through a blind process. Instead of looking at an applicant’s resumé through the usual prism of schools, diplomas and past company employers, the first wave of screening can be done based purely on abilities and achievements.
Other packages also enable the employer to write blind recruiting ads, depicting job descriptions that do away with key phrases and words that are associated with a particular demographic—masculine-implied words such as “driven”, “adventurous”, or “independent”, and those that are feminine-coded such as “honest”, “loyal”, and “interpersonal”.
eLearning Case Studies
Companies are now attempting to make diversity and inclusion—from entry-level employees to the executive suite—hallmarks of their corporate culture. With an objective to identify and address unconscious bias in all processes and behaviors, companies can introduce unconscious bias training curriculum for first-line managers, by calling on eLearning companies for their eLearning courseware and content.
Confronting Hiring Bias in a Virtual Reality Environment
Virtual Reality (VR) technology can further boost unintended hiring bias. In a simulated setting, the user manipulates an avatar that was able to assume any number of demographics for applicants in the hiring process. Based on the gender or ethnicity of the avatar, the user experiences bias during question and answer sessions. The solution would use an immersive VR environment, a diverse collection of avatars, and sample scenarios to pinpoint to participants where bias is demonstrated and understood.
To Infinity and Beyond!
Vamsi Nekkanti looks at the future of data centers – in space and underwater
Data centers can now be found on land all over the world, and more are being built all the time. Because a lot of land is already being utilized for them, Microsoft is creating waves in the business by performing trials of enclosed data centers in the water.
They have already submitted a patent application for an Artificial Reef Data Center, an underwater cloud with a cooling system that employs the ocean as a large heat exchanger and intrusion detection for submerged data centers. So, with the possibility of an underwater cloud becoming a reality, is space the next-or final-frontier?
As the cost of developing and launching satellites continues to fall, the next big thing is combining IT (Information Technology) principles with satellite operations to provide data center services into Earth orbit and beyond.
Until recently, satellite hardware and software were inextricably linked and purpose-built for a single purpose. With the emergence of commercial-off-the-shelf processors, open standards software, and standardized hardware, firms may reuse orbiting satellites for multiple activities by simply downloading new software and sharing a single spacecraft by hosting hardware for two or more users.
This “Space as a Service” idea may be used to run multi-tenant hardware in a micro-colocation model or to provide virtual server capacity for computing “above the clouds.” Several space firms are incorporating micro-data centers into their designs, allowing them to analyze satellite imaging data or monitor dispersed sensors for Internet of Things (IoT) applications.
HPE Spaceborne Computer-2 (a set of HPE Edgeline Converged EL4000 Edge and HPE ProLiant machines, each with an Nvidia T4 GPU to support AI workloads) is the first commercial edge computing and AI solution installed on the International Space Station in the first half of 2021 (Image credit: NASA)
Advantages of Space Data Centers
The data center will collect satellite data, including images, and analyze it locally. Only valuable data is transmitted down to Earth, decreasing transmission costs, and slowing the rate at which critical data is sent down.
The data center might be powered by free, abundant solar radiation and cooled by the chilly emptiness of space. Outside of a solar flare or a meteorite, there would be a minimal probability of a natural calamity taking down the data center. Spinning disc drives would benefit from the space environment. The lack of gravity allows the drives to spin more freely, while the extreme cold in space helps the servers to handle more data without overheating.
Separately, the European Space Agency is collaborating with Intel and Ubotica on the PhiSat-1, a CubeSat with AI (Artificial Intelligence) computing aboard. LyteLoop, a start-up, seeks to cover the sky with light-based data storage satellites.
NTT and SKY Perfect JV want to begin commercial services in 2025 and have identified three primary potential prospects for the technology.
The first, a “space sensing project,” would develop an integrated space and earth sensing platform that will collect data from IoT terminals deployed throughout the world and deliver a service utilizing the world’s first low earth orbit satellite MIMO (Multiple Input Multiple Output) technology.
The space data center will be powered by NTT’s photonics-electronics convergence technology, which decreases satellite power consumption and has a stronger capacity to resist the detrimental effects of radiation in space.
Finally, the JV is looking into “beyond 5G/6G” applications to potentially offer ultra-wide, super-fast mobile connection from space.
The Challenge of Space-Based Data Centers
Of course, there is one major obstacle when it comes to space-based data centers. Unlike undersea data centers, which might theoretically be elevated or made accessible to humans, data centers launched into space would have to be completely maintenance-free. That is a significant obstacle to overcome because sending out IT astronauts for repair or maintenance missions is neither feasible nor cost-effective! Furthermore, many firms like to know exactly where their data is housed and to be able to visit a physical site where they can see their servers in action.
While there are some obvious benefits in terms of speed, there are also concerns associated with pushing data and computing power into orbit. In 2018, Capitol Technology University published an analysis of many unique threats to satellite operations, including geomagnetic storms that cripple electronics, space dust that turns to hot plasma when it reaches the spacecraft, and collisions with other objects in a similar orbit.
The concept of space-based data centers is intriguing, but for the time being-and until many problems are worked out-data centers will continue to dot the terrain and the ocean floor.
Elite Teams recover Systems from Failures in No time (MTTR)
Credits: Published by our strategic partner Kaiburr
Effective Teams in a right environment under Transformative Leadership by and large achieves goals all the time, innovates consistently, resolves issues or fixes problems quickly.
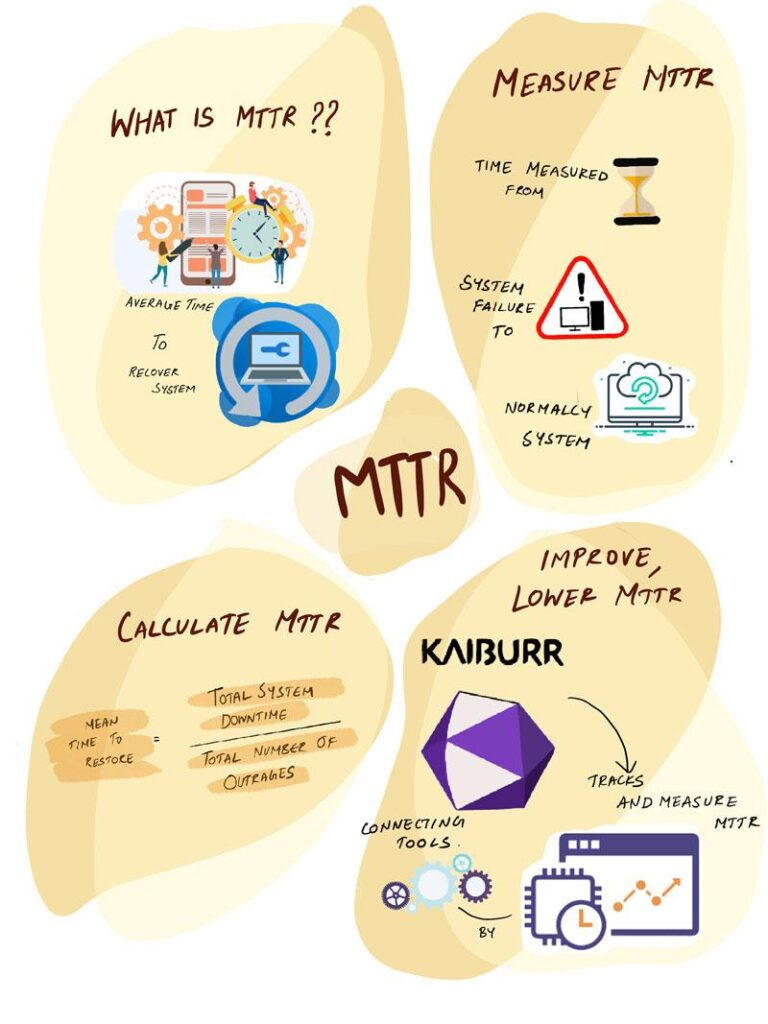
DevOps is to primarily improve Software Engineering practices, Culture, Processes and build effective teams to better serve and delight the Users of IT systems. DevOps focuses on productivity by Continuous Integration and Continuous Deployment (CI-CD) to effectively deliver services with speed and improve Systems Reliability.
The productivity of a System is higher with high performance teams and slower with low performance teams. High performance teams are more agile and highly reliable. We can have better insights on Team performance by measuring Metrics.
DORA (DevOps research and assessment) with their research on several thousands of software professionals across wide geographic regions had come up with their findings that the Elite, High performance, medium and low performance can be differentiated by just the four metrics on Speed and Stability.
The metric ‘Mean time to restore(MTTR) ‘, is the average time to restore or recover the system to normalcy from any production failures. Improving on MTTR, Our teams become Elite and reduces the heavy cost of System downtime.
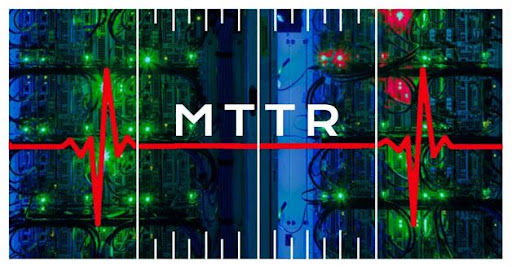
Measure MTTR
MTTR is the time measured from the moment the System fails to serve the Users or other Systems requests in the most expected way to the moment it is brought back to normalcy for the System’s intended response.
The failure of the System could be, because of semantic errors in the new features or new functions or Change requests deployed, memory or integration failures, malfunctioning of any physical components, network issues, External threats(hacks) or just the System Outage.
The failure of the running system against its intended purpose is always an unplanned incident and its restoration to normalcy in the least possible time depends on the team’s capability and its preparedness. Lower MTTR values are better and a higher MTTR value signifies an unstable system and also the team’s inability to diagnose the problem and provide a solution in less time.
MTTR doesn’t take into account the amount of time and resources the teams spend for their preparedness and the proactive measures but its lower value indirectly signifies teams strengths, efforts and Savings for the Organization. MTTR is a measure of team effectiveness.
As per CIO insights, 73% say System downtimes cost their Organization more than $10000/day and the top risks to System availability are Human error, Network failures, Software Bugs, Storage failures and Security threats (hacks).
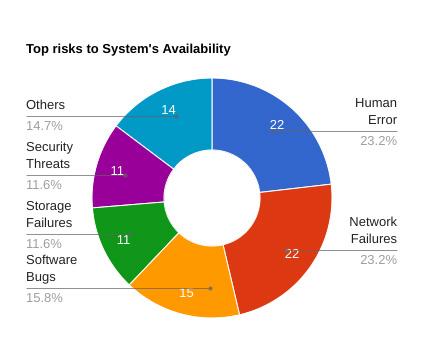
How to Calculate MTTR
We can use a simple formula to calculate MTTR.
MTTR, Mean time to restore = Total Systems downtime / total no. of Outages.
If the System is down for more time, MTTR is obviously high and it signifies the System might be newly deployed, complex, least understood or it is an unstable version. A system down for more time and more frequently causes Business disruptions and Users dissatisfaction. MTTR is affected by the team’s experience, skills and the tools they use. A highly experienced, right skilled team and the right tools they use helps in diagnosing the problem quickly and restoring it in less time. Low MTTR value signifies that the team is very effective in restoring the system quickly and that the team is highly motivated, collaborates well and is well led in a good cultured environment.
Well developed, elite teams are like the Ferrari F1 pit shop team, just in the blink of an eye with superb preparedness, great coordination and collaboration, they Change tyres, repairs the F1 Car and pushes it into the race. MTTR’s best analogy is the time measured from the moment the F1 Car comes into the pit shop till the moment it is released back onto the F1 track. All the productivity and Automation tools our DevOps teams use are like the tools the F1 pitstop team uses.
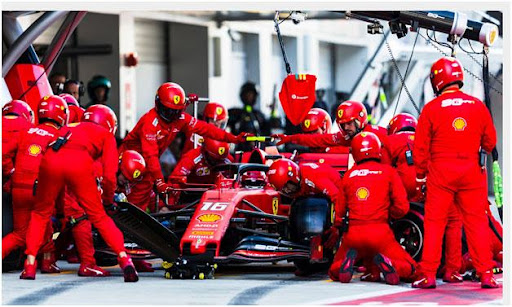
How to improve, lower MTTR
Going with the assumption that a System is stable and still the MTTR is considerably high then there is plenty of room for improvement. In the present times of AI, we have the right tools and DevOps practices to transform teams to high performance and Systems to lower MTTR. Reports of DORA says high performance teams are 96x faster with very low mean time to recover from downtime.
It seems they take very less time, just a few minutes to recover the System from failures than others who take several days. DevOps teams that had been using Automation tools had reduced their costs at least by 30% and lowered MTTR by 50%. The 2021 Devops report says 70% of IT organizations are stuck in the low to mid-level of DevOps evolution.
Kaiburr’s AllOps platform helps track and measure MTTR by connecting to tools like JIRA, ServiceNow, Azure Board, Rally. You can continuously improve your MTTR with near real time views like the following

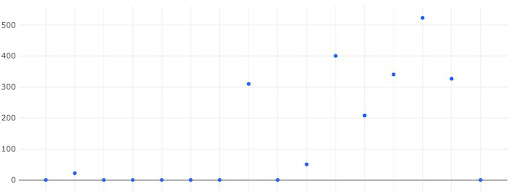
You can also track and measure other KPIs, KRIs and metrics like Change Failure Rate, Lead Time for Changes, Deployment Frequency. Kaiburr helps software teams to measure themselves on 350+ KPIs and 600+ Best Practices so they can continuously improve every day.
Reach us at marketing@sifycorp.com to get started with metrics driven continuous improvement in your organization.
Credits: Published by our strategic partner Kaiburr
Visit DevSecOps – Sify Technologies to get valuable insights
AI and human existence: The ABCs of ADLs
Explained: How even the simplest of day-to-day activities are deeply influenced by ML & AI. Will it become a life-changer?
On any given day, a regular human being does several activities which are performed ritually and without fail. From cleaning to eating and drinking and finding our way across our environments, human life involves many activities that are deemed Activity of Daily Living, or ADL. We have performed these activities in the past without any technological help or advice. Throughout human history, man has invented many a tool to improve the execution of these ADLs, and our current age is no different. Technologies such as ‘Machine Learning’ and ‘Artificial Intelligence’ have enabled many advancements to our lifestyle. Some assist us, however there a few that we need to be cautious about.
Let us stride through these ADLs one by one by following a simple activity timeline of a person’s one-day life as we discover how Machine Learning is shaping human life.
Morning – 5 AM to 10 AM
A day for us typically starts with several activities. Brushing, eating, washing, etc., are a few morning routines that we follow in our day-to-day life.
A day begins by waking up from sleep. Nowadays smart devices such as wrist-fit bands, and smartwatches tell us more about our sleep. These devices take leverage of AI and Machine Learning to provide accurate results with improvement. They help us to improve our sleep quality and behavior which in turn improves our health.
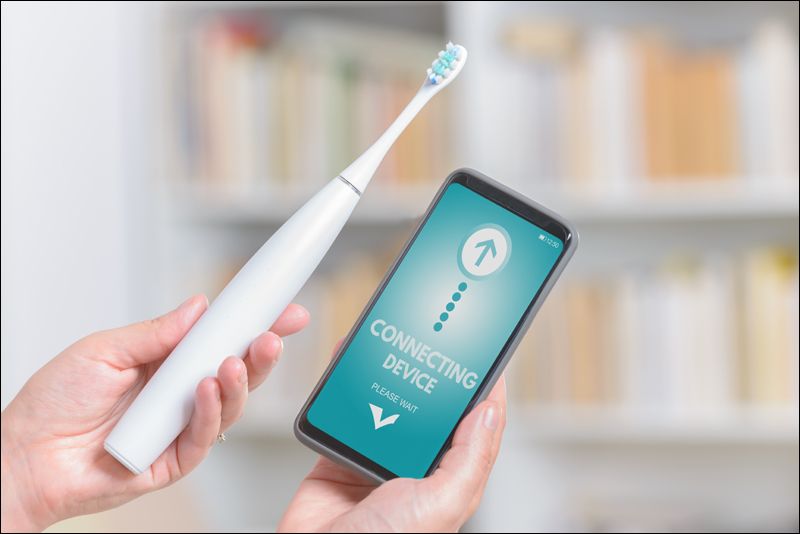
After waking up, we essentially brush our teeth to maintain hygiene and dental wellness. Even in this activity with the help of novel smart wrist devices and smart toothbrushes which use AI and Machine Learning, we study and measure our hand movements, direction, speed, etc., that improve the quality of our brushing which in turns keep our oral health in check. An example of such an application is Oral B Genius X.
Nowadays, washing our hands regularly to maintain hygiene and immunity is very important especially given the COVID scenario around the globe. Many kinds of research have been made to take advantage of the technological development to help in monitoring hand hygiene and give a quality assessment to an individual. Many privatized hospitals have tied up with several industries to implement a smart solution for providing hand hygiene quality assessment. The doctors from these hospitals take advantage of it daily to improve their hygiene and their patients’ as well. An example of such an application is The MedSense Clear system by the MIT Medlab Alumni.
Physical health and maintaining shape have become very underrated due to the new awareness around mental health and its importance. Nevertheless, staying in shape is a very important aspect of people’s lives as it indirectly constitutes mental well-being. Diet planning and eating healthy is something that must be taken care of. With the help of smart mobile and computer applications, we nowadays plan our diet efficiently. With the rise of ‘Machine Learning’, this system is scrutinized, and further research is being conducted to find solutions for problems such as people’s preferences in their eating habits to provide an even better solution.
Mid-Day Activities – 10 AM to 3 PM
Mid-day activities constitute a very wide range of tasks. We regularly use map applications to commute to a certain location. These applications use AI and Machine Learning extensively to provide the best route possible by predicting traffic and other obstacles well before we commute. These applications suggest the best means of transport and the best route to take, they even track and alert us on breakdown of transport services. Examples of such applications are Google Maps, Apple Maps, etc.
Many people work in closed environments either in the office or at home. We are always sedentary, and desk bound. It becomes inevitable to take breaks and go for a short walk and stretch ourselves. And, equally important is to keep ourselves hydrated. With the help of smart devices, we can track the amount of time that we sit continuously. They help us to take a break or even correct our posture if required. Some also help us stay hydrated and suggest improvements based on the environment and atmosphere quality around us. Examples of such devices are Apple Watch, Mi Band, etc.
Evening Activities – 3 PM to 8 PM
Evenings are when we generally try to relax after work and indulge in leisure activities that differs from individual to individual. These activities involve a lot of AI and Machine Learning as it takes advantage of our data right from our preferences to our recent practices. These technology-driven applications and systems need to be handled with utmost caution. We all use the e-commerce facility extensively as it helps us to reduce the time and energy to buy a product as it enables us to shop from wherever we are. This has huge benefits. But we sometimes are ignorant and innocent about the implications that might come. Few applications read our technology usage colossally as they keep track of us more than we know. They improve their recommendation system using this data with the help of AI and Machine Learning and suggest products well before we want to search. If we are not using an official or a recognized application, we are at risk of a privacy breach with the history of our shopping data being stolen or hacked.
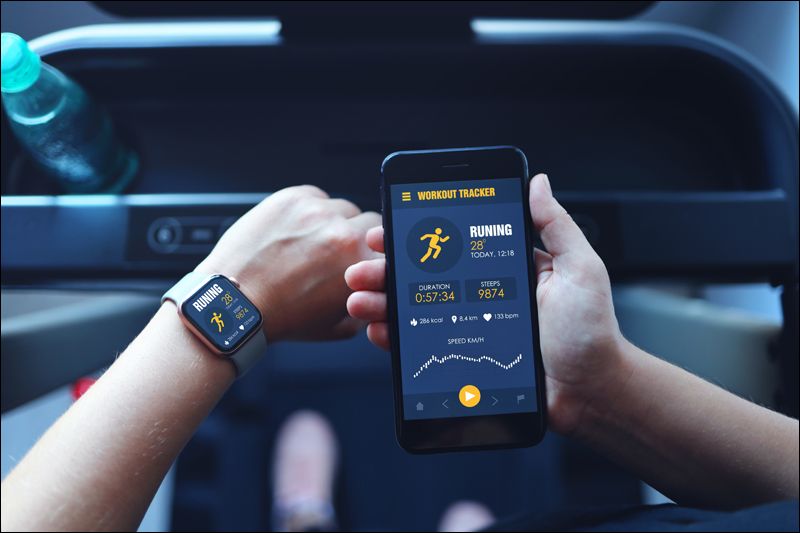
Social media applications have invaded our lives. We connect via different platforms to share, exchange ideas and also to relax. However, some of these applications hold sensitive data about us. These applications have plenty of recommendation systems that are constantly updated to feed posts enjoyable to us. But are we compromising on the sensitive data like our name, address, phone number, etc., as well as our preferences that reveal who we are while doing so? An imminent danger to watch out for here is ‘data leakage’. Some of these applications never encode or cipher our passwords. Other activities during the evening include working out, which a few people prefer in the morning too. With the prevailing COVID situation, we have restricted using the gym frequently. Many applications and systems have been created to assist us to work from home. With the help of AI and Machine Learning, they streamline our workout routine.
End Day Activities – 8 PM to 5 AM
End-day activities help us unwind as we call it a day. We perform certain activities as mentioned previously like eating, brushing, washing, etc. Some smart devices assist us by providing an alarm to indicate our sleeping time. This heavily depends upon technology as it tracks our previous sleep history to let us know in what areas we need to improve. These applications help us learn from our sleep pattern, like how much time we spent in deep sleep and so on. This system heavily uses AI and other sensors to read our breathing, heartbeat and measures those accurately to provide insights. Many wristwatches and fit bands provide this feature.
As we get to the end of it, these applications help us save energy and time as well as lead an enriching life.Having said that, we must also be cautious regarding their influence on us. Take precautions and double-check the application for privacy policies. Always use trusted applications, instead of randomly selecting one that might store unwanted cookies to store sensitive data which might lead to an imminent threat. So, what’s your ADL?
In case you missed: